Monte Carlo Simulation Software For Apple Mac Pc; Free Monte Carlo Simulation Software; Use R software to program probabilistic simulations, often called Monte Carlo simulations. Use R software to program mathematical simulations and to create novel mathematical simulation functions. @RISK by Palisade Corporation is a risk analysis application. SimVoi ® Monte Carlo Simulation Add-in for Excel For Mac Excel 2016-2019-365 and Windows Excel 2010-2013-2016-2019-365 SimVoi provides random number generator functions as inputs for your model, automates Monte Carlo simulation, computes value of information, and displays the results. Video OmniPlan; Monte Carlo Simulation. See how Monte Carlo Simulations work, and how you can use them in OmniPlan to plan your projects better. The simulation uses the well know Monte Carlo method, in which some of the variables affecting the electron trajectory are randomly selected from a specific distribution of values. Based on the simulation of a large number of electrons, it is possible to have a picture of the electron beam's behaviour inside the specimen under investigation. Monte Carlo simulation enables us to model situations that present uncertainty and then play them out on a computer thousands of times. Note: The name Monte Carlo simulation comes from the computer simulations performed during the 1930s and 1940s to estimate the probability that the chain reaction needed for an atom bomb to detonate would work successfully.
The XLSTAT-Monte Carlo Simulations module for XLSTAT allows you to create models with assessed risk in Microsoft Excel and uses simulation methods such as Monte Carlo and Latin Hypercubes simulations to estimate the distribution (including confidence intervals) of important variables.
XLSTAT-Monte Carlo Simulations module is a key decision making tool for people working on statistical risk analysis of models which may contain uncertain values. These uncertainties can be expressed through more than 30 distributions.
For example, in a financial model for establishing a budget, the sales volume of a product is not certain, but we can estimate that it should be between two bounds, A and B, with a most likely value M. This can be statistically represented by a triangular distribution. The total revenue for all products is a sum of triangular distributions. XLSTAT-Sim can produce in mere seconds, an estimated distribution of the revenue, its median, average and a 95% confidence interval.
Note on XLSTAT-Monte Carlo Simulations: The Sim module runs under all Windows versions of Excel, but not on the Mac.
Demo version
A trial version of XLSTAT-Monte Carlo Simulations is included in the main XLSTAT download.
Prices and ordering
These analyses are included in the XLStat-Forecast, XLSTAT-Marketing, XLSTAT-Quality and XLStat-Premium packages.
The simulation methods available in XLSTAT-Monte Carlo Simulations are Monte Carlo and Latin Hypercubes.
Simulation models
Simulation models allow to obtain information, such as mean or median, on variables that do not have an exact value, but for which we can know, assume or compute a distribution. If some 'result' variables depend of these 'distributed' variables by the way of known or assumed formulae, then the 'result' variables will also have a distribution. XLSTAT-Monte Carlo Simulations allows you to define the distributions, and then obtain through simulations an empirical distribution of the input and output variables as well as the corresponding statistics.
Simulation models are used in many areas such as finance and insurance, medicine, oil and gas prospecting, accounting, or sales prediction.
Four elements are involved in the construction of a simulation model:
- Distributions are associated to random variables. XLSTAT gives a choice of more than 20 distributions to describe the uncertainty on the values that a variable can take. For example, you can choose a triangular distribution if you have a quantity for which you know it can vary between two bounds, but with a value that is more likely (a mode). At each iteration of the computation of the simulation model, a random draw is performed in each distribution that has been defined.
- Scenario variables allow to include in the simulation model a quantity that is fixed in the model, except during the tornado analysis where it can vary between two bounds.
- Result variables correspond to outputs of the model. They depend either directly or indirectly, through one or more Excel formulae, on the random variables to which distributions have been associated and if available on the scenario variables. The goal of computing the simulation model is to obtain the distribution of the result variables.
- Statistics allow to track a given statistic a result variable. For example, we might want to monitor the standard deviation of a result variable.
A correct model should comprise at least one distribution and one result. Models can contain any number of these four elements.
Options for simulation models
A model can be limited to a single Excel sheet or can use a whole Excel folder.
Simulation models can take into account the dependencies between the input variables described by distributions. If you know that two variables are usually related such that the correlation coefficient between them is 0.4, then you want that, when you do simulations, the sampled values for both variables have the same property. This is possible in XLSTAT-Monte Carlo Simulations.
Perform sensitivity analysis through random parameter variation
Monte Carlo simulation is a technique used to study how a model responds to randomly generated inputs. It typically involves a three-step process:
- Randomly generate “N” inputs (sometimes called scenarios).
- Run a simulation for each of the “N” inputs. Simulations are run on a computerized model of the system being analyzed.
- Aggregate and assess the outputs from the simulations. Common measures include the mean value of an output, the distribution of output values, and the minimum or maximum output value.
Systems analyzed using Monte Carlo simulation include financial, physical, and mathematical models. Because simulations are independent from each other, Monte Carlo simulation lends itself well to parallel computing techniques, which can significantly reduce the time it takes to perform the computation.
The MATLAB® language provides a variety of high-level mathematical functions you can use to build a model for Monte Carlo simulation and to run those simulations. MATLAB is used for financial modeling, weather forecasting, operations analysis, and many other applications.
In financial modeling, Monte Carlo Simulation informs price, rate, and economic forecasting; risk management; and stress testing. Financial Toolbox™ provides stochastic differential equation tools to build and evaluate stochastic models. Risk Management Toolbox™ facilitates credit simulation, including the application of copula models.
For more control over input generation, Statistics and Machine Learning Toolbox™ provides a wide variety of probability distributions you can use to generate both continuous and discrete inputs.
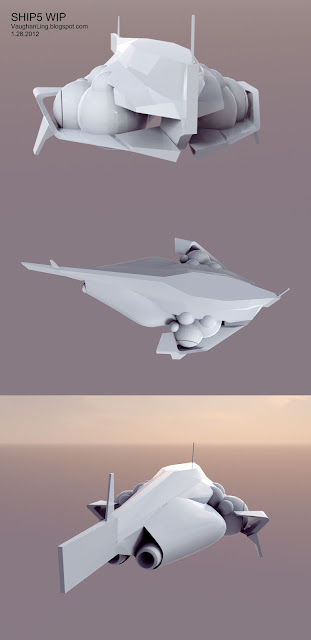
Monte Carlo Simulation Formula
You can model and simulate multidomain systems in Simulink® to represent controllers, motors, gains, and other components. The design and testing of these complex systems involves multiple steps, including identifying which model parameters have the greatest impact on requirements and behavior, logging and analyzing simulation data, and verifying the system design.
Monte Carlo simulations help you gain confidence in your design by allowing you to run parameter sweeps, explore your design space, test for multiple scenarios, and use the results of these simulations to guide the design process through statistical analysis. Simulink Design Optimization™ provides interactive tools to perform this sensitivity analysis and influence your Simulink model design.
To improve the performance of your Monte Carlo simulations, you can distribute the computations to run in parallel on multiple cores using Parallel Computing Toolbox™ and MATLAB Parallel Server™.
MATLAB Examples and How To
- Sequential Blackjack - Example
- Pricing American Basket Options by Monte Carlo Analysis - Example
- Monte Carlo Analysis of a PK/PD Model for an Antibacterial Agent - Example
- Simulating Dependent Random Variables Using Copulas - Example
- Developing and Implementing Scenario Analysis Models to Measure Operational Risk - User Story
- Monte-Carlo Simulations & Robustness Analysis - File Exchange
Simulink Examples and How To
Monte Carlo Simulation Vs Machine Learning
- Design Exploration Using the Sensitivity Analysis Tool (5:01) - Video
- Robustness Analysis for Monte Carlo Simulations - Example
- Improving System Models Using Monte Carlo Techniques - AIAA Paper
- PID Tuning with Reference Tracking and Plant Uncertainty - Example
- Run Monte Carlo Simulations in Parallel - Example
Software Reference
- Monte Carlo Simulation of Conditional Variance Models - Documentation
- uss: Specify uncertain state-space models or convert LTI model to uncertain state-space model - Function
- simsd: Simulate linear models using Monte Carlo method - Function
- Credit Simulation Using Copulas - Documentation
- Sensitivity Analysis Using Monte Carlo Simulations in Simulink - Documentation
- parsim: Directly run multiple parallel simulations from the parsim command - Function
See also: formal verification, financial engineering, random number, system verification and validation, Monte Carlo simulation in computational finance, parameter estimation, load forecasting, modeling and simulation, simulation software, Monte Carlo simulation videos, predictive modeling